Deploying YOLOv5 on NVIDIA Jetson Orin with cuDLA: Quantization-Aware Training to Inference
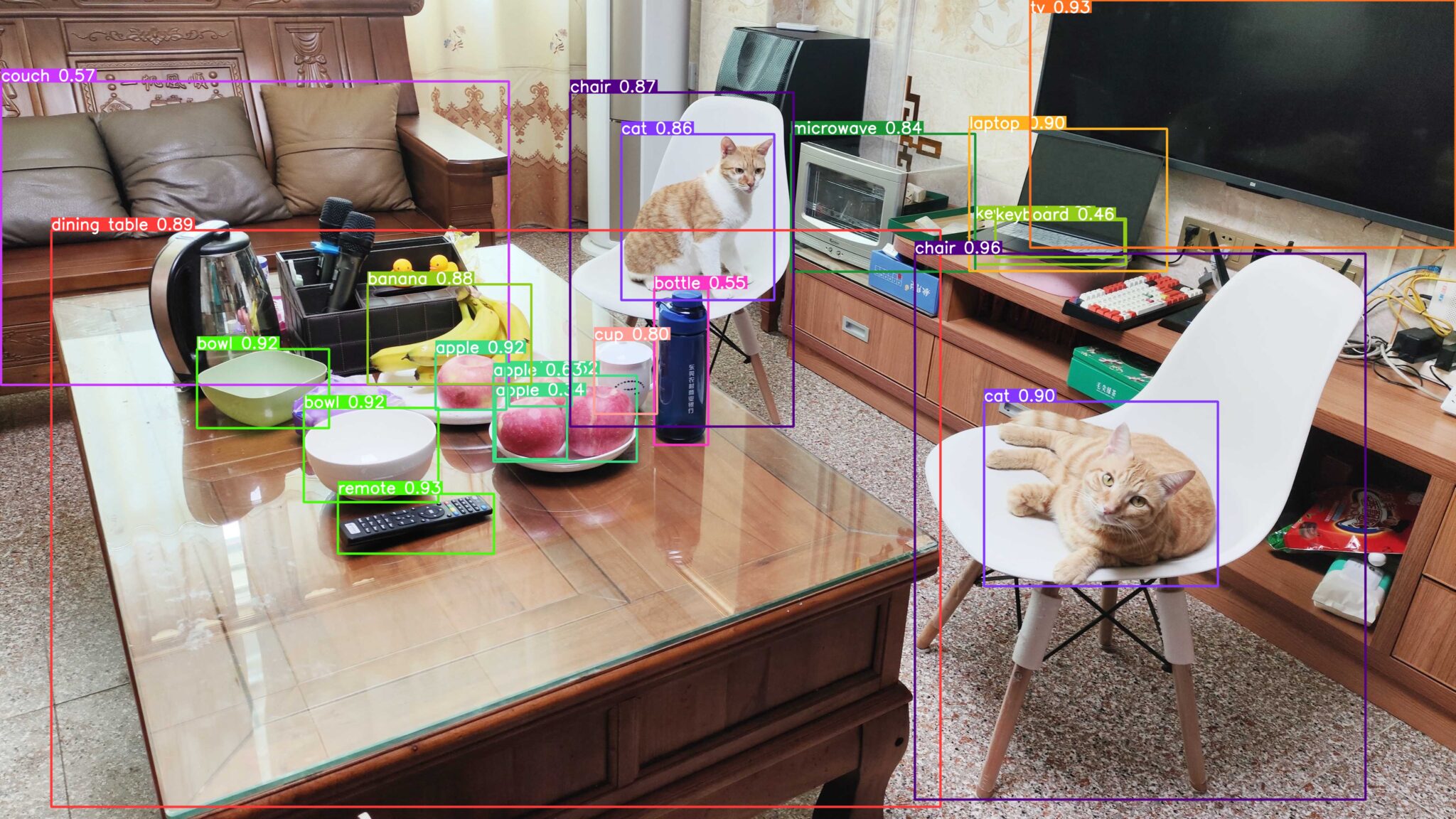
Deploying YOLOv5 on NVIDIA Jetson Orin with cuDLA
Introduction
This sample demonstrates how to deploy the YOLOv5 object detection network on the NVIDIA Jetson Orin platform using the cuDLA library. We use Quantization-Aware Training (QAT) to achieve a balance between inference performance and accuracy. The model is trained on the COCO dataset and achieves a Mean Average Precision (mAP) of 37.3 with DLA INT8, which is close to the official FP32 mAP of 37.4.
QAT Training and Export for DLA
To optimize the YOLOv5 model for inference on Jetson Orin, we apply Quantization-Aware Training (QAT). This involves quantizing the model to INT8 precision while minimizing the loss in accuracy. We also create a custom quantization module for DLA to ensure compatibility.
Q/DQ Translator Workflow
The Q/DQ Translator is used to translate the QAT-trained ONNX graph to an ONNX model without Q/DQ nodes and with PTQ tensor scales. The quantization scales are extracted from the Q/DQ nodes in the QAT model. This ONNX model, along with the PTQ calibration cache file, can be used by TensorRT to build a DLA engine.
Deploying Network to DLA for Inference
We deploy the network and run inference using CUDA through TensorRT and cuDLA. This provides an interface for DLA loadable building and integration with the GPU. We can run DLA tasks in hybrid mode, where they are submitted to a CUDA stream for seamless synchronization with other CUDA tasks. Alternatively, we can use standalone mode to save resources if there is no CUDA context in the pipeline.
Conclusion
By deploying YOLOv5 on Jetson Orin with cuDLA, we can achieve high-performance object detection with minimal loss in accuracy. The QAT training and export process ensures compatibility with DLA, and the cuDLA library provides seamless integration with CUDA for efficient inference.