RAG 101: Demystifying Retrieval-Augmented Generation Pipelines
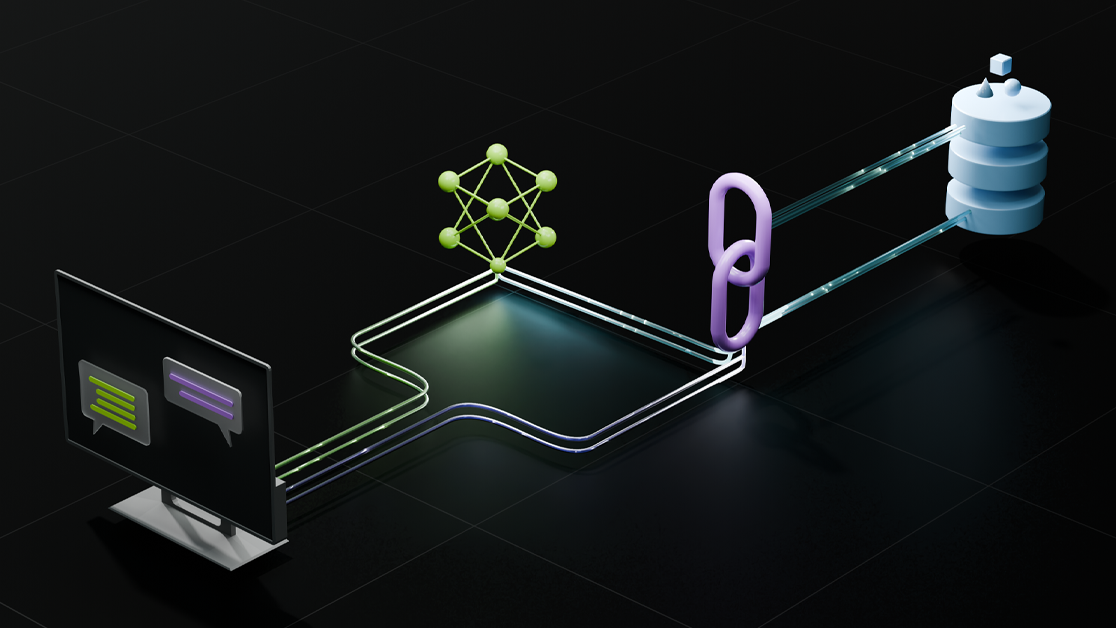
RAG 101: Demystifying Retrieval-Augmented Generation Pipelines
This article explores the concept of retrieval augmented generation (RAG) and its benefits for enterprises. RAG involves augmenting large language models (LLMs) with business data, enabling them to comprehend and generate human-like responses. By incorporating real-time data access, RAG empowers LLM solutions with up-to-date information, reducing the likelihood of hallucinations. It also preserves data privacy and improves user experiences. The RAG pipeline consists of document pre-processing, ingestion, and embedding generation, as well as an inference pipeline for user queries and response generation. Document ingestion involves loading diverse data from various sources, while generating embeddings represents the data in numerical format to facilitate efficient processing. The processed data and embeddings are stored in vector databases, ensuring quick access during real-time interactions. LLMs utilize the stored information to generate relevant responses based on user queries. By adopting RAG techniques, enterprises can enhance their AI applications by providing accurate and proprietary information, increasing user trust, and minimizing hallucinations.